The Next Wave of AI Innovation: Breakthroughs Shaping the Future
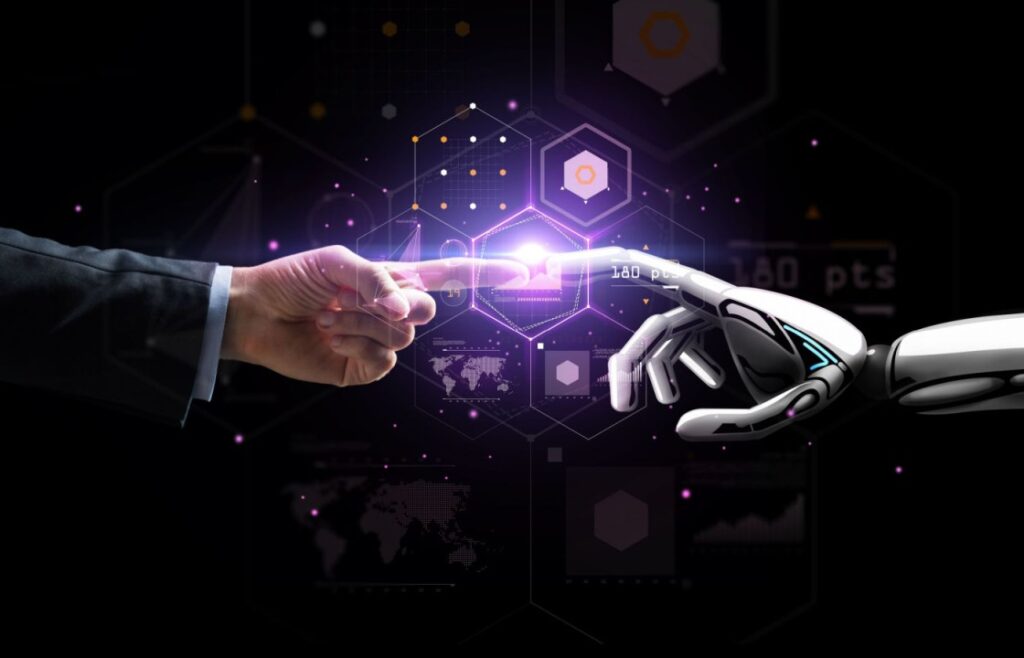
Artificial Intelligence (AI) is rapidly evolving, with groundbreaking innovations pushing the boundaries of what machines can achieve. Researchers and engineers are developing more advanced deep learning models, efficient AI algorithms, and novel applications that are transforming industries. As AI continues to mature, we are witnessing significant progress in natural language processing (NLP), reinforcement learning, AI-driven drug discovery, and explainable AI (XAI).
This article explores some of the most exciting AI research innovations happening today, the challenges researchers face, and how these developments are shaping the future of artificial intelligence.
1. Advances in Natural Language Processing (NLP): The Rise of Larger Language Models
One of the most impressive areas of AI research in recent years has been natural language processing (NLP). With the development of large-scale transformer-based models, AI has made significant strides in understanding and generating human-like text.
- GPT-3 by OpenAI: One of the most powerful language models to date, GPT-3, has revolutionized text generation. With 175 billion parameters, it is capable of writing essays, summarizing articles, translating languages, and even generating code. This marks a major leap forward from its predecessor, GPT-2, and has raised discussions about AI’s ability to pass the Turing Test.
- BERT & Contextual Understanding: Google’s BERT (Bidirectional Encoder Representations from Transformers) has enhanced AI’s ability to process text in a context-aware manner, significantly improving search engine results and question-answering systems.
While these advancements are impressive, they also come with challenges, such as bias in AI-generated text, high computational costs, and concerns over misinformation. Researchers are actively working on improving ethical AI practices to make NLP models safer and more reliable.
2. Reinforcement Learning: AI That Learns from Experience
Reinforcement learning (RL) has been one of the most exciting research areas in AI, allowing systems to learn from trial and error and improve their decision-making over time.
- DeepMind’s AlphaFold: One of the most groundbreaking achievements in RL is AlphaFold, an AI system that predicts protein structures with atomic accuracy. This has enormous implications for drug discovery, medical research, and biology, as understanding protein folding is crucial for fighting diseases.
- AI in Robotics: Reinforcement learning is making robots more adaptable, allowing them to learn new tasks without being explicitly programmed. Companies like Boston Dynamics and OpenAI are pushing the limits of AI-driven robotics for applications in manufacturing, automation, and logistics.
- Game-Playing AI Models: AI models trained with RL, such as DeepMind’s AlphaStar, have demonstrated superhuman-level strategies in real-time games like StarCraft II, showing AI’s potential in complex decision-making environments.
Despite these advancements, training reinforcement learning models is computationally expensive, and real-world applications require fine-tuning to deal with unpredictable environments. However, continued research is making RL more accessible and practical for industry applications.
3. AI-Driven Drug Discovery: Revolutionizing Healthcare
AI is making a major impact in drug discovery and biomedical research, accelerating the development of new treatments. Traditional drug discovery methods are time-consuming and costly, but AI is reducing the timeline from years to months.
- AI and COVID-19 Drug Development: AI-powered models have helped researchers identify potential COVID-19 treatments by analyzing vast datasets of chemical compounds. Companies like DeepMind, BenevolentAI, and Insilico Medicine have leveraged AI to assist in drug repurposing efforts.
- Predicting Molecular Interactions: AI models are capable of simulating how different molecules interact, making it easier to design new drugs with higher precision. This is paving the way for more personalized medicine and targeted therapies.
- AI in Medical Diagnostics: Deep learning models are being used to detect diseases from medical images, such as identifying early-stage cancer from radiology scans with accuracy comparable to human doctors.
AI’s role in healthcare is growing, but regulatory and ethical considerations must be addressed to ensure AI-driven medical solutions are safe, unbiased, and clinically validated.
4. Explainable AI (XAI): Making AI Decisions More Transparent
As AI systems become more powerful, a growing concern is their lack of interpretability. Many deep learning models function as “black boxes,” making it difficult to understand how they arrive at their decisions.
- The Importance of Explainability: Businesses and regulators are demanding more transparent AI models, especially in critical sectors like finance, healthcare, and criminal justice. If an AI model denies a loan application or diagnoses a disease, it is crucial to understand why it made that decision.
- XAI Techniques: Researchers are developing methods to make AI more explainable, such as SHAP (Shapley Additive Explanations) and LIME (Local Interpretable Model-Agnostic Explanations), which help users understand AI model predictions.
- Ethical AI & Bias Reduction: Addressing bias in AI is a major focus of explainability research. Governments and AI research organizations are working on ethical AI guidelines to ensure models are fair, transparent, and accountable.
By improving AI explainability, researchers aim to build trustworthy and interpretable models that can be safely deployed in real-world scenarios.
5. The Challenges and Future of AI Research
While AI research is advancing rapidly, several challenges remain:
- Data Privacy Concerns: AI models require large amounts of data, raising concerns about how user data is collected, stored, and used.
- High Computational Costs: Training massive models like GPT-3 requires huge amounts of computing power, making AI research energy-intensive and costly.
- Regulatory Uncertainty: Governments are still developing policies around AI ethics, accountability, and deployment. Striking a balance between innovation and regulation will be crucial.
Looking ahead, AI research will focus on making models more efficient, ethical, and applicable to real-world problems. Innovations in AI hardware, federated learning, and hybrid AI models will further expand AI’s potential across industries.
Final Thoughts: AI Research is Entering a New Era
The progress in AI research over the past few years has been nothing short of revolutionary. From large-scale language models and reinforcement learning to AI-powered drug discovery and explainable AI, these advancements are shaping the future of artificial intelligence.
As researchers continue to push the boundaries of AI, businesses, governments, and society must work together to ensure responsible AI deployment. The next decade will define how AI integrates into our world, and the innovations happening today will serve as the foundation for the intelligent systems of tomorrow.
One thing is certain—AI research is evolving faster than ever, and its impact on humanity is just beginning.