Exploring the Next Frontier: Breakthroughs in AI Research and Emerging Innovations
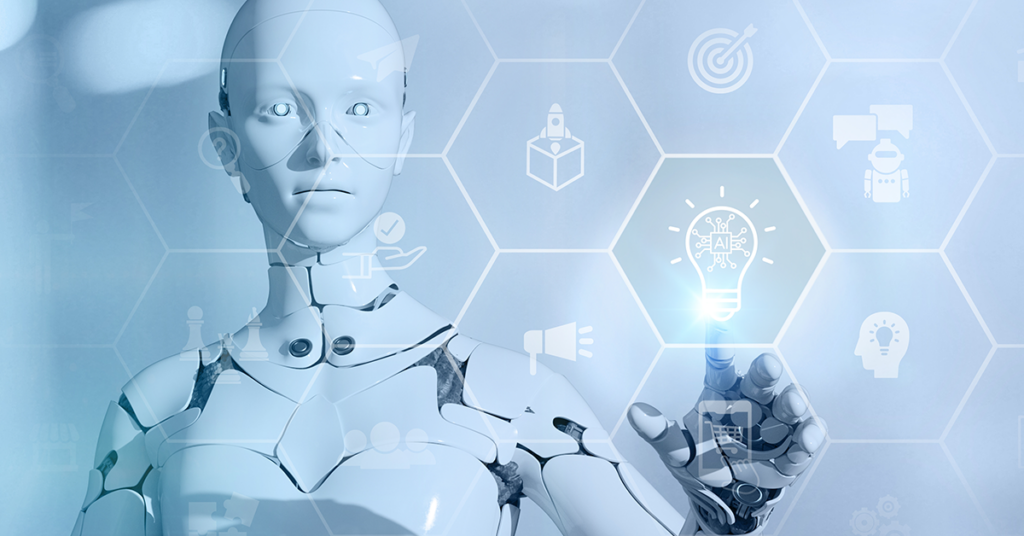
Artificial Intelligence (AI) research is advancing at an astonishing pace, pushing the boundaries of what machines can accomplish. From self-learning algorithms to autonomous decision-making systems, recent breakthroughs are setting the stage for the next wave of technological evolution. Researchers are refining AI’s ability to understand, adapt, and optimize solutions across various fields, enabling smarter automation, advanced problem-solving, and deeper insights into complex data.
As AI continues to evolve, its integration into scientific research, engineering, and real-world applications is becoming more profound. While much of the conversation around AI focuses on its commercial use, a significant portion of AI research is aimed at tackling some of the world’s most pressing scientific and technological challenges. This article delves into some of the latest innovations in AI research, highlighting new techniques, groundbreaking applications, and the challenges that come with these advancements.
1. Self-Supervised Learning: The Future of Machine Intelligence?
Traditional machine learning models require large, labeled datasets to learn effectively. However, labeling vast amounts of data is costly and time-consuming, creating a bottleneck in AI development. Self-supervised learning (SSL) is emerging as a powerful technique that enables AI models to learn from raw, unlabeled data, mimicking the way humans learn from experience rather than explicit instruction.
A. The Potential of Self-Supervised Learning
- SSL allows AI to recognize patterns, classify information, and generate insights without the need for extensive human-labeled data.
- It is particularly useful in domains where labeled data is scarce, such as medical research, space exploration, and climate science.
- AI models trained using SSL can generalize better across different datasets, making them more adaptable and efficient.
B. Real-World Applications
- Computer Vision: SSL is being used to train image recognition models on vast collections of unlabeled images, improving AI’s ability to detect objects and interpret scenes.
- Healthcare: AI is being trained to analyze medical scans, pathology slides, and genetic sequences with minimal human supervision, accelerating diagnosis and treatment discovery.
- Autonomous Systems: Self-supervised learning is helping robotics and self-driving cars develop a deeper understanding of their environment without relying solely on manually labeled datasets.
SSL represents a major shift in AI research, offering a glimpse into a future where AI models learn more independently, requiring less human intervention.
2. AI-Powered Scientific Discovery: A New Era of Research
AI is proving to be an invaluable tool in scientific research, where its ability to process massive datasets, simulate complex systems, and identify patterns is accelerating discoveries across multiple disciplines.
A. AI in Physics and Materials Science
- AI-driven models are being used to simulate atomic interactions, helping researchers discover new materials for energy storage, electronics, and sustainable construction.
- Quantum computing, when combined with AI, is allowing scientists to model molecular structures and optimize materials for better performance and efficiency.
- AI-powered tools are being employed to analyze data from particle accelerators and deep-space observations, helping physicists test hypotheses more efficiently.
B. AI in Drug Discovery and Biomedical Research
- AI algorithms are accelerating drug discovery by predicting how molecules interact with biological systems, cutting down the time required for experimental trials.
- AI-powered tools like AlphaFold are solving the protein folding problem, a breakthrough that could lead to new treatments for genetic and neurodegenerative diseases.
- Researchers are using AI to model disease progression and treatment responses, allowing for more personalized medicine and targeted therapies.
These advancements demonstrate that AI is not just automating existing processes but also enabling entirely new ways of conducting scientific research.
3. Generative AI and Its Expanding Role in Innovation
Generative AI, powered by deep learning models, is not only transforming creative industries but also playing a vital role in engineering, design, and synthetic data generation.
A. AI in Engineering and Design
- AI-powered design algorithms are optimizing product development, enabling engineers to create stronger, lighter, and more efficient materials and structures.
- Generative models are assisting in automated architecture design, offering novel solutions for energy-efficient and sustainable buildings.
- AI is being used in aerospace engineering to develop more aerodynamic designs, reducing fuel consumption and improving overall efficiency.
B. AI in Data Generation and Simulation
- AI-generated synthetic data is helping researchers train machine learning models when real-world data is scarce or sensitive.
- AI-driven simulation environments allow businesses and researchers to test new concepts before implementation, reducing risk and cost.
- Generative AI is also being used in robotics, where simulated environments allow AI models to train without real-world testing.
As AI becomes more sophisticated, generative models are opening entirely new possibilities in research, creativity, and problem-solving.
4. Ethical and Computational Challenges in AI Research
With great power comes great responsibility. As AI research advances, ethical concerns, computational limitations, and transparency issues become more pressing.
A. The Ethics of AI-Generated Content
- AI-generated text, images, and videos raise concerns about misinformation, deepfakes, and manipulation.
- Researchers are developing AI-detection tools to distinguish between real and AI-generated content, ensuring accountability in media and communication.
- AI ethics frameworks are being proposed to address bias, fairness, and responsible AI deployment.
B. The Environmental Cost of AI
- Training large AI models requires significant computational resources, leading to high energy consumption and carbon emissions.
- Researchers are exploring ways to make AI training more efficient, including low-power neural networks and energy-efficient computing hardware.
C. The “Black Box” Problem and Explainability
- Many AI models operate as black boxes, making it difficult to understand how decisions are made.
- Explainable AI (XAI) techniques aim to provide clearer insights into AI reasoning, making AI systems more transparent and accountable.
By addressing these challenges, AI research can continue pushing the boundaries of innovation while ensuring responsible and ethical development.
Final Thoughts: AI’s Expanding Role in the Future of Innovation
The landscape of AI research is evolving rapidly, offering breakthroughs that go beyond automation and into realms of scientific discovery, creativity, and innovation. From self-supervised learning and generative AI to scientific discovery and explainable models, AI is shaping the future of research in ways never seen before.
As researchers continue to refine AI’s capabilities, it is crucial to balance progress with responsibility, ensuring that AI remains a force for good in solving some of the world’s biggest challenges. The future of AI is not just about making machines smarter; it’s about making AI a collaborative tool that enhances human ingenuity and expands the frontiers of what’s possible.