Bridging the Gap Between AI and Scientific Discovery
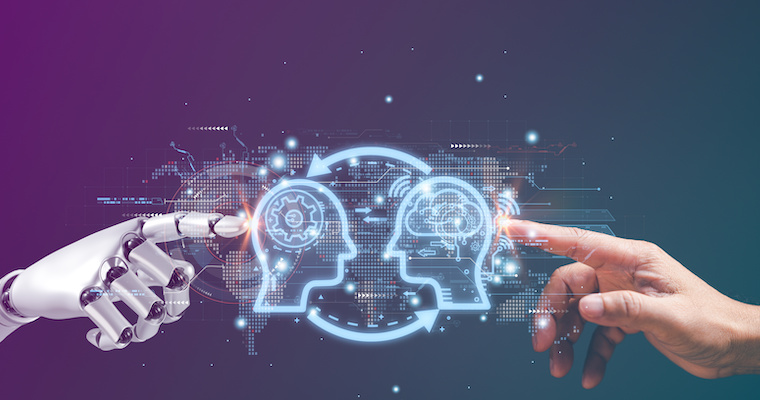
Scientific discovery has traditionally been a labor-intensive process, requiring meticulous experimentation, years of data collection, and collaboration among experts from various disciplines. While technology has always played a role in accelerating research, the integration of artificial intelligence (AI) is now fundamentally reshaping how discoveries are made.
AI is no longer just a tool for automation; it has evolved into a cognitive partner that can process vast amounts of data, identify patterns invisible to the human eye, and even generate hypotheses. From physics to biomedicine, AI-driven research tools are enabling breakthroughs that would have been impossible using conventional methods alone.
This article explores the transformative role of AI in accelerating scientific discovery, its applications across multiple fields, and the challenges that must be addressed to ensure AI’s responsible and effective use in research.
AI as a Catalyst for Discovery
Scientific research has always relied on trial and error, hypothesis-driven experimentation, and statistical modeling. However, AI is revolutionizing this process by enabling researchers to test thousands of variables simultaneously, model complex systems with unparalleled accuracy, and extract meaningful insights from unstructured data.
1. The Role of AI in Hypothesis Generation
- AI models trained on scientific literature, experimental data, and real-world observations can propose new research questions and guide scientists toward unexplored areas of inquiry.
- Natural language processing (NLP) models analyze vast archives of research papers, helping scientists identify gaps in knowledge and propose new theories.
- AI-powered simulations allow researchers to test potential outcomes before conducting physical experiments, reducing costs and time spent on trial-and-error methods.
2. AI in Data Processing and Pattern Recognition
- Scientific datasets, whether in genomics, climate science, or astrophysics, are often too vast and complex for traditional computational methods.
- AI algorithms, particularly deep learning models, excel at identifying hidden patterns and correlations that might be overlooked by human researchers.
- AI is enhancing research reproducibility by reducing human bias in data interpretation and ensuring consistency across experiments.
By augmenting human intelligence with AI-driven pattern recognition, researchers are accelerating the pace of discovery and making sense of increasingly complex datasets.
Applications of AI in Scientific Research
The impact of AI-driven discovery is being felt across multiple fields, revolutionizing how scientists approach some of the world’s most pressing challenges.
1. AI in Drug Discovery and Biomedical Research
- AI is transforming the drug development process, which traditionally takes years of testing and billions of dollars in investment.
- Machine learning models analyze chemical structures and biological interactions to predict which compounds have the highest potential for therapeutic use.
- AI-driven platforms like AlphaFold have revolutionized protein structure prediction, allowing researchers to understand how diseases operate at a molecular level.
- Personalized medicine is being enhanced by AI, which helps doctors tailor treatments to individual genetic profiles, improving outcomes and minimizing side effects.
2. AI in Climate Science and Environmental Research
- AI-powered climate models are enhancing predictions of extreme weather events, allowing for better preparedness and disaster response.
- Machine learning algorithms analyze satellite imagery to track deforestation, pollution levels, and biodiversity loss in real time.
- AI-driven simulations are optimizing renewable energy grid management, improving the efficiency of wind and solar power distribution.
- Predictive models are helping scientists assess the long-term impacts of climate change, guiding global policy decisions.
3. AI in Physics and Materials Science
- AI is playing a crucial role in discovering new materials with unique properties, aiding in the development of stronger, lighter, and more energy-efficient materials.
- In particle physics, AI is processing massive datasets from particle accelerators, helping researchers identify previously unknown subatomic particles.
- AI-driven quantum simulations are pushing forward research in quantum mechanics, superconductors, and nanotechnology.
These applications highlight AI’s growing role in addressing complex scientific challenges, allowing for faster and more precise breakthroughs.
Challenges and Ethical Considerations
Despite its immense potential, AI-driven scientific discovery comes with significant challenges that must be addressed.
1. The Risk of AI-Generated Errors
- AI models can generate false positives or misleading correlations if trained on incomplete or biased datasets.
- Scientific research must incorporate human oversight to validate AI-generated insights before applying them in real-world scenarios.
2. Data Privacy and Ethical Use of AI
- AI-driven biomedical research involves sensitive patient data, requiring strict adherence to privacy laws and ethical guidelines.
- Ensuring that AI systems do not discriminate based on genetic, racial, or socioeconomic factors is essential for ethical AI in healthcare and research.
3. The Challenge of Explainability
- Many AI models function as black boxes, meaning their decision-making processes are not always transparent.
- Scientists need to develop explainable AI (XAI) frameworks to ensure that research conclusions can be understood, trusted, and replicated.
4. Computational and Energy Costs
- Training complex AI models requires substantial computing power, contributing to high energy consumption.
- Researchers must balance the environmental impact of AI with its scientific benefits, developing more energy-efficient algorithms and hardware.
Addressing these challenges is crucial for integrating AI into the scientific community in a way that is responsible, transparent, and beneficial to all.
The Future of AI in Scientific Research
As AI continues to evolve, its role in scientific discovery is expected to expand even further. Key developments to watch include:
- AI-Powered Autonomous Labs: Fully automated research facilities where AI conducts experiments, analyzes results, and refines hypotheses without human intervention.
- Enhanced AI-Human Collaboration: AI will increasingly act as a research assistant, proposing ideas and helping scientists explore new theories.
- AI-Driven Theoretical Science: AI models may soon be capable of formulating entirely new scientific theories, pushing beyond human intuition and computational constraints.
- Interdisciplinary AI Research: AI’s role will extend beyond individual fields, facilitating cross-disciplinary discoveries in medicine, physics, and environmental science.
These advancements will shape the next generation of scientific breakthroughs, leading to discoveries at a pace never before seen.
Final Thoughts: A New Era of Discovery
AI is no longer just a tool for automation—it is becoming a driving force behind scientific discovery, changing the way researchers approach some of the world’s most complex problems. By combining human expertise with AI-driven insights, scientists are unlocking new possibilities in medicine, climate science, physics, and beyond.
However, as AI takes on a greater role in shaping scientific advancements, the importance of ethical guidelines, transparency, and responsible AI development cannot be overstated. Ensuring that AI-driven discoveries are reliable, explainable, and beneficial to society will be key to realizing AI’s full potential in research.
With the right approach, AI will not only accelerate scientific progress—it will redefine how we explore, understand, and shape the world around us.