The Breakthrough in AI-Generated Protein Structures
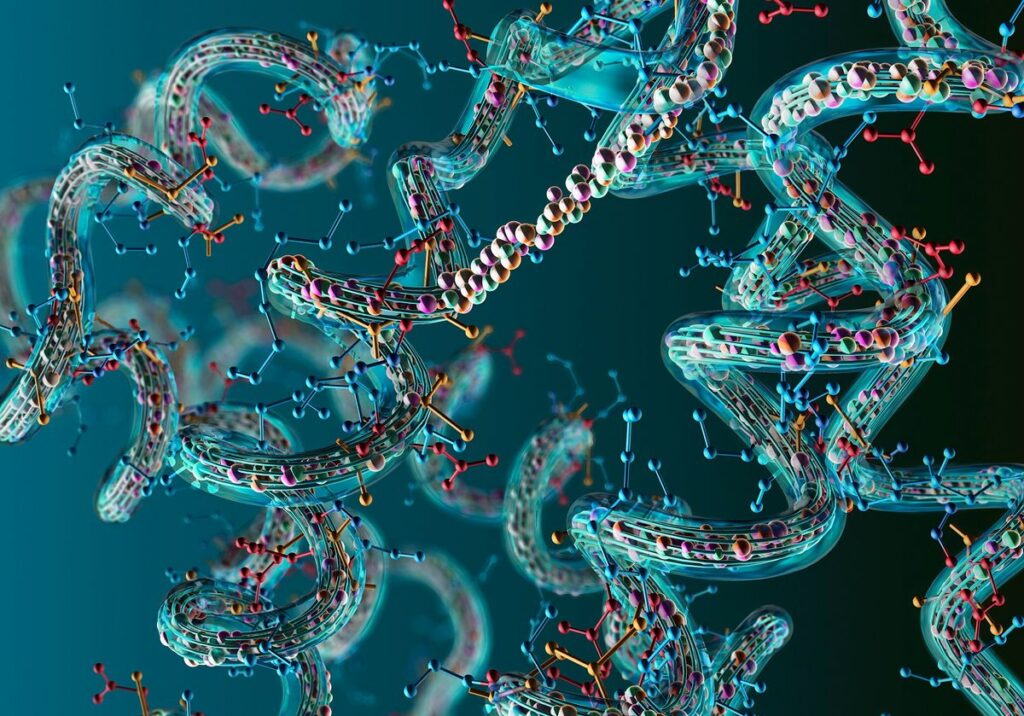
For decades, one of the biggest challenges in molecular biology has been accurately predicting the three-dimensional structure of proteins. Understanding these structures is crucial for drug discovery, disease treatment, and advancements in biotechnology. However, determining protein structures through traditional methods such as X-ray crystallography and cryo-electron microscopy has been an expensive, time-consuming process—sometimes taking years for a single protein.
This landscape dramatically changed in June 2022, when DeepMind announced a major expansion of its AlphaFold AI model. AlphaFold, which had already stunned the scientific community in 2021 by solving the protein-folding problem, was now capable of predicting the structures of nearly every known protein in the human body and thousands of other species. This marked one of the most significant AI-driven breakthroughs in life sciences, accelerating research that could lead to new treatments for diseases, sustainable bioengineering solutions, and innovations in synthetic biology.
The AlphaFold Expansion: What Changed?
Prior to this announcement, AlphaFold had already demonstrated its ability to accurately predict protein structures with unprecedented precision, providing a database of over 350,000 protein structures. In June 2022, DeepMind took this even further, releasing a dataset of over 200 million protein structures, covering nearly every protein known to science.
This advancement meant that:
- Researchers worldwide could access AI-generated protein structures instantly, without needing to conduct costly and time-consuming lab experiments.
- The dataset included proteins from plants, bacteria, fungi, and animals, opening new possibilities in agriculture, medicine, and industrial biotechnology.
- Scientists working on rare diseases and previously unexplored proteins now had a roadmap for developing targeted drugs and therapies.
This was not just an improvement—it was a game-changer, making structural biology as accessible as searching for an image on the internet.
Why This Matters for Science and Medicine
The implications of this AI-driven breakthrough extend across multiple disciplines, offering new opportunities in drug discovery, synthetic biology, and evolutionary research.
1. Faster Drug Discovery and Development
- Understanding protein structures helps design drugs that fit precisely into molecular targets, improving treatment effectiveness.
- AI-generated protein models allow pharmaceutical companies to reduce years of research into months, expediting the discovery of new treatments.
- AlphaFold has already been used to develop new antibiotics and cancer drugs, with even more potential applications now possible.
2. Advancements in Genetic and Rare Disease Research
- Many genetic disorders are caused by misfolded proteins. Having access to detailed models helps researchers understand why these misfoldings occur and how to fix them.
- Scientists studying orphan diseases (conditions that affect small populations and have limited research funding) now have the tools to analyze unknown proteins without the need for costly lab experiments.
3. Sustainable Agriculture and Food Innovation
- Proteins play a key role in crop resilience, soil health, and food production. Understanding plant proteins at a molecular level can lead to drought-resistant crops and improved agricultural yields.
- AI-driven protein modeling could lead to the creation of lab-grown meat with improved texture and nutritional value, reducing reliance on livestock farming.
4. Bioengineering and Industrial Applications
- AlphaFold’s predictions could help design new enzymes for biofuels, biodegradable plastics, and waste management.
- Synthetic biology companies are now using AI-generated protein models to engineer bacteria that can break down pollutants or produce bio-based chemicals.
These applications highlight how AI is no longer just a tool for research—it is actively shaping the future of medicine, agriculture, and industry.
Challenges and Ethical Considerations
While AlphaFold’s expansion is widely celebrated, it also raises new questions and challenges.
1. Ensuring Accuracy and Reliability
- AI-generated protein models, while highly accurate, still require experimental validation before being used in critical applications such as drug development.
- Some rare or highly flexible proteins might not be fully represented in AI predictions, requiring further refinement of these models.
2. Open-Source vs. Proprietary Data
- DeepMind made AlphaFold’s protein structures freely available, but concerns remain about how AI-driven discoveries will be used in pharmaceutical patents and commercial applications.
- If private companies start limiting access to AI-driven protein models, it could slow down global research efforts.
3. Ethical and Biosecurity Risks
- With AI-generated protein structures, it is now easier than ever to synthesize and manipulate biological molecules. While this is beneficial for medicine, it could also be misused in bioterrorism or unethical genetic modifications.
- Governments and regulatory bodies need to develop frameworks to ensure AI-driven biological research is used responsibly.
Addressing these challenges will be key to maximizing the benefits of AI in molecular biology while minimizing risks.
The Road Ahead: What’s Next for AI in Protein Research?
As AlphaFold and similar AI models continue to evolve, the future of AI-driven structural biology holds several exciting possibilities:
- AI-designed proteins for new medicines: Instead of just predicting existing protein structures, AI could help scientists design entirely new proteins for targeted therapies.
- AI-powered enzyme discovery: AI models could rapidly identify natural enzymes that break down plastics, process industrial waste, or improve food production.
- Quantum computing and AI integration: As quantum computing advances, it could be combined with AI to simulate complex protein interactions in real-time, revolutionizing material science and medicine.
This breakthrough has set a new standard for AI in scientific discovery, demonstrating how machine learning is no longer just assisting human researchers—it is accelerating progress in ways that were unimaginable just a decade ago.
Final Thoughts: AI’s Role in the Future of Life Sciences
The expansion of AlphaFold in June 2022 represents a milestone in AI’s contribution to scientific research. By providing accurate protein structures at an unprecedented scale, this technology is not just changing how research is done—it is redefining what is possible in life sciences.
With open access to 200 million+ protein structures, scientists around the world now have an AI-powered foundation to develop cures, engineer sustainable materials, and push the boundaries of biotechnology. However, as with any powerful technology, it is essential to balance innovation with ethical responsibility, ensuring AI-driven discoveries benefit humanity as a whole.
The next phase of AI in biology is just beginning, and what comes next could be even more transformative.