Breakthrough in AI-Powered Scientific Discovery Speeds Up Research
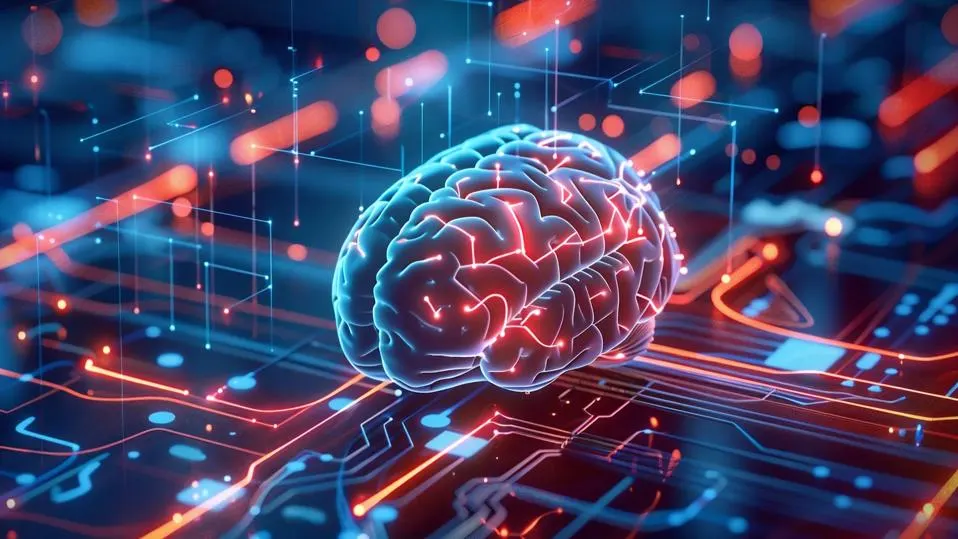
Scientific research has always relied on meticulous experimentation, data analysis, and hypothesis testing, often taking years to yield groundbreaking discoveries. However, in February 2024, AI-driven research models reached a new level of sophistication, enabling scientists to accelerate breakthroughs in physics, chemistry, and biomedical sciences.
With advancements in self-learning AI models, autonomous research assistants, and AI-driven simulations, researchers began leveraging AI to identify patterns in massive datasets, optimize experiments, and even generate new scientific theories. These tools weren’t just assisting scientists—they were actively reshaping how discoveries were made, reducing trial-and-error cycles, and enhancing data-driven hypothesis generation.
How AI Was Transforming Scientific Research
Traditionally, researchers depended on manual data collection, statistical modeling, and laboratory-based experimentation, which often required extensive resources and time. AI-powered research tools introduced a radical shift in the scientific process by enabling:
- AI-driven hypothesis generation – Machine learning models analyzed existing research and proposed novel scientific hypotheses based on uncovered patterns.
- Autonomous laboratory experiments – AI-controlled robotic systems conducted experiments at unprecedented speed, refining methods based on real-time feedback.
- AI-enhanced simulations and modeling – Advanced AI-powered computational tools simulated chemical reactions, climate patterns, and biological processes, reducing reliance on physical trials.
With these capabilities, scientific research was evolving into a highly efficient, AI-augmented process that accelerated the pace of innovation.
Fields Rapidly Adopting AI in Research
AI-powered research tools were being integrated across multiple scientific disciplines, where data-driven analysis and experimental optimization were critical.
1. AI in Biomedical Research and Drug Discovery
- AI-driven models helped predict protein structures and molecular interactions, allowing researchers to develop targeted drug therapies faster than ever before.
- AI-assisted clinical trial simulations modeled how new drugs would behave in human systems, minimizing reliance on extensive live testing.
2. AI in Climate Science and Environmental Research
- AI-powered models analyzed global weather patterns, predicting extreme climate events with higher accuracy.
- AI-enhanced simulations optimized renewable energy solutions, improving efficiency in solar, wind, and battery technologies.
3. AI in Theoretical Physics and Quantum Computing
- AI-driven computational models assisted in exploring new quantum physics theories, revealing potential advancements in quantum computing.
- Autonomous AI researchers simulated particle interactions, accelerating discoveries in subatomic physics.
These applications signified a shift in how fundamental scientific questions were being approached, with AI serving as a key collaborator in discovery.
Challenges and Ethical Considerations in AI-Powered Research
Despite its potential, AI-driven research presented key challenges that the scientific community had to address.
1. Reliability and Reproducibility of AI-Generated Findings
- AI-generated hypotheses and experimental results needed to be validated through independent replication, ensuring scientific integrity.
- Scientists worked to refine AI transparency, ensuring AI-generated insights were interpretable and testable.
2. Ethical Concerns in AI-Led Research
- AI models trained on biased datasets could introduce unintended distortions in scientific conclusions, leading to flawed discoveries.
- Researchers debated the role of AI in sensitive areas like genetic engineering and autonomous laboratory decision-making, ensuring ethical safeguards.
3. The Future Role of Human Researchers
- As AI took on more responsibility in the research process, concerns arose about how human scientists would collaborate with AI-driven systems.
- The focus shifted toward developing AI models that enhanced, rather than replaced, human intuition and expertise.
These challenges highlighted the importance of maintaining scientific rigor while embracing AI-powered efficiency.
What’s Next for AI in Scientific Discovery?
With AI continuing to evolve, the next wave of scientific advancements was expected to include:
- AI-powered autonomous research labs – Fully AI-operated laboratories would conduct continuous, data-driven experiments, optimizing research productivity.
- AI-driven scientific collaboration networks – AI models would analyze global research findings, helping scientists connect interdisciplinary insights for groundbreaking discoveries.
- Quantum AI for complex simulations – The combination of AI and quantum computing would unlock previously impossible simulations in physics, chemistry, and biology.
These developments positioned AI as a transformative force in the future of scientific innovation.
A Defining Moment for AI in Research
The integration of AI-powered scientific discovery tools in February 2024 marked a turning point in how research was conducted, reducing time-consuming processes and opening new frontiers in multiple fields. AI was no longer just a tool for data analysis—it was an active participant in the research process, generating ideas, testing hypotheses, and accelerating knowledge creation.
However, as AI became more involved in scientific exploration, ensuring ethical oversight, maintaining transparency, and preserving scientific integrity remained critical challenges.