Advancements in AI: The Impact of CLIP on Computer Vision and Multimodal Learning
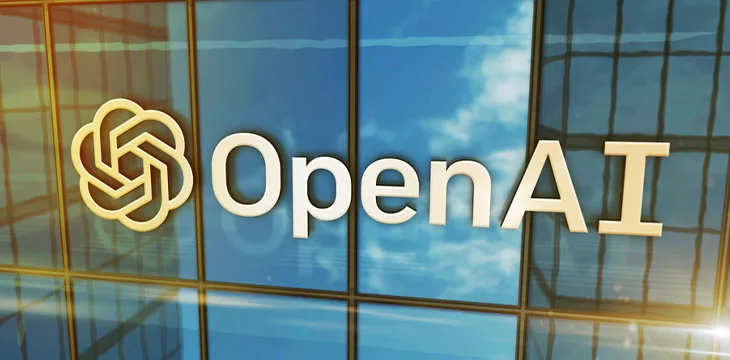
Artificial Intelligence (AI) is constantly evolving, pushing the limits of what machines can understand and create. Recent breakthroughs in deep learning have allowed AI systems to interpret language, generate realistic images, and analyze complex datasets with increasing accuracy. One of the most promising developments in AI research has been the rise of multimodal learning, where models can process and relate information across different formats—such as text, images, and audio—simultaneously.
A major step forward in this space has been the development of CLIP (Contrastive Language–Image Pretraining) by OpenAI. CLIP is an AI model capable of understanding images through natural language without requiring extensive labeled datasets. Unlike traditional image recognition systems that rely on rigid classification categories, CLIP can analyze and describe images in an open-ended way, making it a powerful tool for a wide range of applications.
In this article, we explore how CLIP works, why it represents a significant advancement in AI, and the potential real-world applications and challenges of multimodal learning.
1. What is CLIP and How Does It Work?
CLIP is an AI model that bridges the gap between computer vision and natural language processing (NLP). It is trained on hundreds of millions of image-text pairs sourced from the internet, allowing it to develop a broad understanding of visual concepts in relation to natural language.
A. Learning Without Labeled Data
Traditional image recognition models require massive labeled datasets like ImageNet, where each image is manually annotated with specific categories. This approach is time-consuming, expensive, and limits models to predefined categories. CLIP, on the other hand, learns in a self-supervised manner, associating images with descriptive text captions.
- Instead of relying on human-defined labels, CLIP is trained using a contrastive learning approach, where it learns to match images with their most relevant textual descriptions.
- This enables the model to recognize a wide range of objects, styles, and abstract concepts without being explicitly trained on them.
B. Zero-Shot Learning: Recognizing New Objects Without Additional Training
A significant advantage of CLIP is its ability to perform zero-shot learning. This means it can classify or describe images it has never seen before by leveraging its knowledge of natural language and visual relationships.
For example, if given an image of an astronaut riding a horse, CLIP can generate a caption such as:
“An astronaut in a white spacesuit riding a brown horse in a desert landscape.”
This level of context-aware visual understanding was previously difficult for AI to achieve without extensive fine-tuning on labeled datasets.
2. Real-World Applications of CLIP
Because CLIP is versatile, efficient, and does not require task-specific training, it has the potential to revolutionize multiple fields.
A. Enhancing Image Search and Content Moderation
- CLIP can power more intelligent image search engines by allowing users to search for images using natural language queries rather than relying on exact keywords or metadata.
- Social media platforms and online marketplaces can use CLIP for automated content moderation, identifying inappropriate or harmful images based on contextual descriptions rather than simple filters.
B. AI-Powered Creativity and Design
- Designers and artists can use CLIP to generate AI-assisted artwork based on textual descriptions. Combined with generative models like DALL·E, CLIP can help create unique and customizable visuals.
- AI-powered photo and video editing tools can leverage CLIP’s contextual understanding to make automated enhancements based on user preferences.
C. Robotics and Autonomous Systems
- CLIP’s ability to interpret visual information through language makes it ideal for robotic vision systems, where robots need to understand their environment based on natural language commands.
- In autonomous vehicles, CLIP could help with scene understanding, allowing cars to make better decisions based on complex, real-world visual inputs.
D. Accessibility and Assistive Technologies
- CLIP could play a major role in helping visually impaired individuals by describing images and surroundings using natural language.
- AI-driven accessibility tools could allow users to interact with visual content through voice commands and text-based queries, making technology more inclusive.
These applications illustrate how multimodal AI models like CLIP can bridge the gap between human communication and machine perception, creating more intuitive and adaptable AI-driven tools.
3. Challenges and Ethical Considerations
While CLIP represents a major step forward in AI, it also comes with technical and ethical challenges that need to be addressed before widespread adoption.
A. Bias in AI Models
- Because CLIP is trained on internet data, it inherits biases present in online content, which can lead to unintended or problematic associations.
- If not properly monitored, AI-generated descriptions could reinforce stereotypes or misinterpret sensitive content, leading to ethical concerns.
B. Explainability and Reliability
- Like many deep learning models, CLIP operates as a black box, meaning that it is difficult to fully understand why it makes certain decisions.
- Ensuring that AI-generated content is trustworthy, fair, and interpretable is a major challenge for researchers and businesses adopting CLIP-based solutions.
C. Misuse and Misinformation
- Advanced AI models capable of generating realistic image descriptions could be misused for deepfake detection evasion, AI-generated misinformation, or automated propaganda.
- Proper regulation and ethical guidelines must be in place to prevent AI technologies from being leveraged for harmful purposes.
Despite these challenges, ongoing research in AI ethics, fairness, and interpretability will help ensure that multimodal models like CLIP are used responsibly and effectively in the real world.
Final Thoughts: A New Era of AI-Driven Perception
CLIP marks a major advancement in AI research, showcasing the potential of multimodal learning and zero-shot image understanding. By enabling machines to connect language and vision in a more flexible and human-like manner, CLIP paves the way for a future where AI-powered tools can seamlessly interact with people, understand context, and process complex visual information.
As researchers continue refining contrastive learning techniques and multimodal AI models, we can expect to see even more sophisticated applications emerge—from next-generation search engines and creative tools to AI-powered robotics and assistive technologies. The development of AI models like CLIP is reshaping the way we interact with machines, pushing the limits of what AI can achieve in understanding and interpreting the world around us.