The Evolution of AI: How Adaptive Systems Are Redefining Intelligence
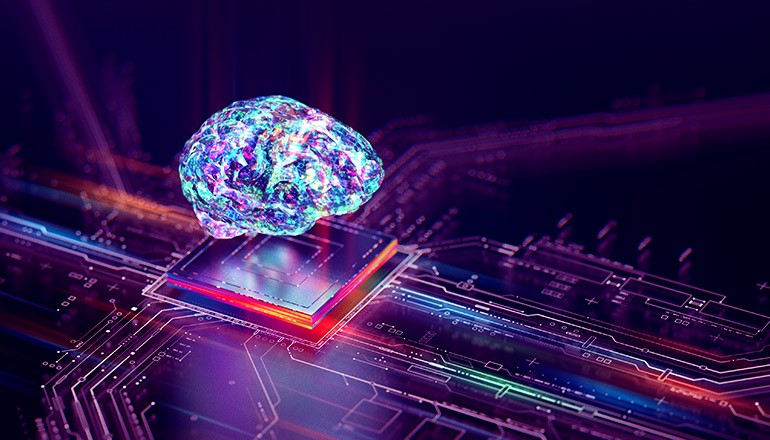
Artificial Intelligence (AI) has rapidly evolved beyond simple automation, moving towards adaptive, self-improving systems capable of learning and evolving over time. Rather than relying solely on pre-programmed rules or static datasets, modern AI systems are increasingly dynamic, context-aware, and capable of adjusting their behavior based on real-time feedback.
This shift is enabling AI to become more efficient across various fields, from healthcare and finance to robotics and autonomous systems. The next generation of AI models is not only making faster predictions but also understanding patterns, adapting to changing environments, and continuously refining its knowledge base.
In this article, we will explore how adaptive AI systems are redefining intelligence, the real-world applications of these self-learning models, and the challenges they present as we move toward more autonomous, decision-making AI.
1. Adaptive AI: Moving Beyond Static Learning Models
Traditional machine learning models require large amounts of labeled data to perform specific tasks. Once trained, these models remain relatively fixed unless manually updated with new data. However, adaptive AI goes beyond this static approach by continuously learning, adjusting, and optimizing performance based on real-world interactions.
A. Reinforcement Learning: AI That Learns Through Trial and Error
One of the most exciting developments in adaptive AI is reinforcement learning (RL), where AI systems learn through experience by interacting with their environment and receiving feedback.
- RL has been widely applied in robotics, game-playing AI, and financial modeling, where decision-making processes must evolve over time.
- Notable examples include DeepMind’s AlphaGo and AlphaZero, which demonstrated how AI can outperform human experts in complex strategic games by self-learning optimal moves.
- RL is also being used in autonomous vehicle navigation, where AI systems learn to make real-time adjustments to driving conditions.
B. Continual Learning: AI That Retains and Expands Knowledge
Unlike conventional AI models that require retraining from scratch when encountering new information, continual learning enables AI to retain past knowledge while integrating new insights.
- This is particularly useful in fields like healthcare and cybersecurity, where AI systems must adapt to emerging threats and evolving medical research.
- Continual learning allows AI to build upon existing knowledge without forgetting past experiences, making it more human-like in its ability to adapt over time.
These advancements are leading to AI models that are not just more accurate but also more flexible and sustainable, capable of improving without constant manual intervention.
2. Real-World Applications of Adaptive AI Systems
The transition from static AI to adaptive, self-learning models is driving innovation across multiple industries. Here are some areas where adaptive AI is making a significant impact:
A. Healthcare: AI That Evolves with Medical Knowledge
- AI-powered diagnostic systems are continuously improving their accuracy by learning from new patient data, medical research, and real-time clinical feedback.
- Adaptive AI is helping drug discovery processes by analyzing vast biochemical datasets and adjusting predictions based on new laboratory findings.
- Personalized medicine is being enhanced through AI models that adapt to individual patient responses, optimizing treatment plans dynamically.
B. Financial Services: Smarter Fraud Detection and Risk Analysis
- AI-driven fraud detection systems are evolving by learning from new fraud patterns and financial transaction behaviors to identify potential threats before they occur.
- Adaptive AI is being used in algorithmic trading, where AI models adjust investment strategies based on real-time market fluctuations.
- Credit scoring models are now incorporating alternative financial data, allowing for more fair and accurate loan assessments.
C. Robotics: Self-Learning Machines in Manufacturing and Automation
- Industrial robots powered by adaptive AI are learning to improve their efficiency on factory floors, adjusting movements and workflows based on real-time operational data.
- AI-powered warehouse management systems are optimizing logistics by predicting inventory demand and supply chain disruptions dynamically.
- In autonomous delivery systems, self-learning AI allows robots to navigate unpredictable environments, adapting their paths based on real-world obstacles.
D. AI in Smart Cities and Infrastructure
- Traffic management systems are using AI-powered predictive analytics to optimize traffic flow and reduce congestion dynamically.
- Adaptive AI is playing a role in energy consumption and grid optimization, allowing smart cities to adjust power distribution based on real-time demand.
- AI-powered public safety monitoring systems are identifying and responding to emerging threats and emergency situations with increasing precision.
These applications illustrate how AI is shifting from static prediction models to dynamic, evolving systems capable of improving their performance over time.
3. Challenges in Implementing Adaptive AI
While the benefits of adaptive AI are vast, they also introduce new challenges in scalability, security, and ethical considerations.
A. Computational Costs and Scalability
- Adaptive AI models require continuous processing power, leading to higher computational costs compared to traditional machine learning models.
- Developing efficient low-power AI solutions is crucial for scaling adaptive AI applications to edge devices and IoT systems.
B. Ethical Concerns and Bias in Adaptive AI
- As AI learns dynamically, it can develop biases based on feedback loops, potentially reinforcing harmful or unintended patterns.
- Without proper oversight, adaptive AI systems could amplify misinformation or manipulate data-driven decisions in unintended ways.
- Developing transparent and explainable AI frameworks is essential to ensuring adaptive AI models remain fair and accountable.
C. Security Risks in Self-Learning Systems
- Adaptive AI models, if not properly monitored, can become vulnerable to adversarial attacks, where malicious actors manipulate AI behavior through deceptive inputs.
- AI systems that autonomously update their knowledge must have safeguards in place to prevent unintended actions based on misleading data.
Addressing these challenges will be critical to ensuring adaptive AI remains a reliable and ethical force in technological advancement.
Final Thoughts: The Road Ahead for Adaptive AI
The shift from static, rule-based AI models to adaptive, self-learning systems represents a paradigm shift in artificial intelligence. By allowing AI to evolve, optimize, and improve autonomously, researchers are creating systems that are not just more intelligent but also more resilient and responsive to real-world challenges.
As industries continue to integrate adaptive AI technologies, businesses and researchers must work together to ensure responsible AI development, maintain transparency, and mitigate potential risks. The future of AI lies in models that can continuously learn, make smarter decisions, and adapt to an ever-changing world—a transformation that is already reshaping the way we think about intelligence itself.
The coming years will define how well society can harness the power of adaptive AI while ensuring that its growth remains ethical, accountable, and beneficial for all.