Understanding the Shift Toward Hybrid AI Systems
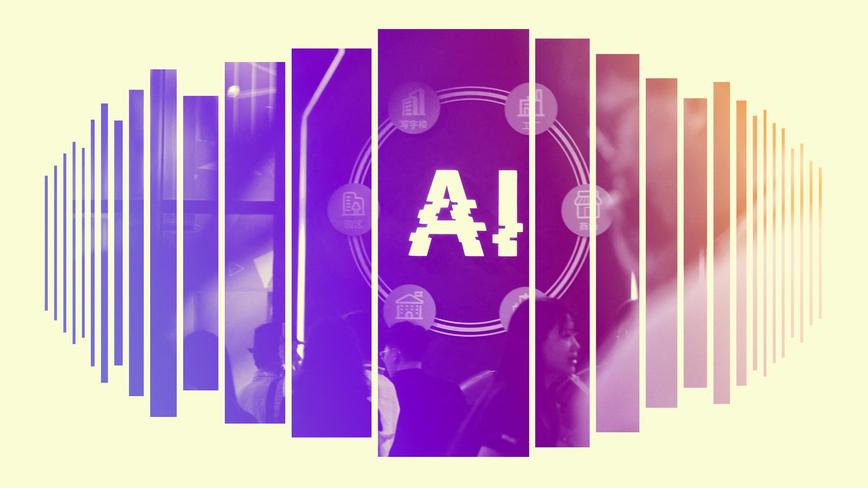
The field of artificial intelligence has traditionally been divided between symbolic AI, which relies on rules and logic, and machine learning-based AI, which learns patterns from data. While machine learning has dominated recent AI advancements, there is growing recognition that combining these two approaches—creating hybrid AI systems—can lead to more robust and adaptable intelligence.
Hybrid AI systems are emerging as a promising solution to the limitations of deep learning, such as its need for massive datasets, lack of explainability, and difficulty in reasoning through abstract or symbolic tasks. By integrating machine learning models with rule-based reasoning, knowledge graphs, and symbolic AI methods, researchers are creating systems that can better understand context, reason through problems, and make decisions with more reliability.
This article explores how hybrid AI is reshaping the way machines process information, make decisions, and interact with humans, its key applications, and the challenges that still need to be addressed.
1. What Is Hybrid AI and Why Is It Important?
Hybrid AI refers to AI systems that combine different forms of intelligence—typically machine learning and symbolic reasoning—to create more flexible, efficient, and interpretable AI models.
A. The Shortcomings of Pure Machine Learning
While deep learning models have achieved impressive results in image recognition, natural language processing, and predictive analytics, they have several fundamental weaknesses:
- Data dependency: Machine learning models require huge amounts of labeled data to generalize well.
- Lack of reasoning: Deep learning models excel at pattern recognition but struggle with logical reasoning and symbolic understanding.
- Explainability issues: Many deep learning models function as “black boxes,” making their decision-making process difficult to interpret and trust.
B. How Hybrid AI Bridges the Gap
Hybrid AI attempts to address these challenges by combining statistical learning with rule-based reasoning and structured knowledge representation. Key techniques include:
- Neuro-symbolic AI: Integrates neural networks with logic-based symbolic AI to improve reasoning and interpretation.
- Knowledge graphs: Helps AI models store and retrieve structured knowledge, improving their ability to understand relationships between concepts.
- Explainable AI (XAI) methods: Enhances interpretability by allowing models to justify their decisions with logical reasoning.
By fusing these methods, hybrid AI creates systems that can adapt to new problems with less data, explain their decisions more clearly, and generalize knowledge across different domains.
2. Key Applications of Hybrid AI Systems
Hybrid AI is already being applied across multiple industries, enhancing AI’s ability to handle complex decision-making and reasoning tasks.
A. AI in Healthcare: Improving Diagnosis and Treatment Plans
- Medical AI systems are integrating deep learning with knowledge graphs to help doctors make more accurate diagnoses by combining pattern recognition with structured medical knowledge.
- Hybrid AI is being used to predict disease progression by incorporating patient history, genetic data, and clinical research insights.
- AI-driven chatbots in healthcare are using rule-based decision trees combined with NLP to provide better patient interactions and medical advice.
B. AI in Legal and Compliance Analysis
- Legal AI tools use hybrid approaches to analyze legal contracts and regulatory documents, extracting important clauses and identifying risks.
- Rule-based AI models are combined with natural language understanding (NLU) techniques to interpret complex legal texts and suggest compliance strategies.
- Hybrid AI assists in automating document reviews, reducing the time required for legal due diligence.
C. AI in Autonomous Systems and Robotics
- Self-driving cars benefit from hybrid AI by combining machine learning-based perception models with rule-based decision-making to handle unpredictable driving conditions.
- Industrial robots are integrating symbolic reasoning with real-time sensory data processing to improve adaptability in manufacturing and logistics.
- Hybrid AI enables smart assistants and robotic process automation (RPA) systems to understand workflows and execute tasks based on predefined logic.
D. AI in Financial Risk Management and Fraud Detection
- Banks are leveraging hybrid AI to detect fraudulent transactions by integrating pattern-based anomaly detection with rule-based risk assessments.
- AI-powered financial advisors are using a mix of deep learning for predictive analytics and symbolic AI for reasoning-based investment strategies.
- Hybrid AI is improving credit risk assessment by incorporating both historical transaction patterns and structured financial regulations.
The ability to combine machine learning with structured reasoning makes hybrid AI a powerful tool for real-world applications, allowing AI models to explain, adapt, and generalize more effectively.
3. Challenges and Considerations in Hybrid AI
While hybrid AI offers promising benefits, there are still challenges to overcome in its development and implementation.
A. Complexity in Model Integration
- Designing hybrid AI systems requires expertise in both deep learning and symbolic reasoning, making implementation more complex than traditional AI models.
- There is still no standard framework for integrating symbolic reasoning with neural networks, leading to inconsistencies in hybrid AI approaches.
B. Computational Costs and Scalability
- Hybrid AI models can be computationally expensive, especially when integrating large-scale deep learning models with structured reasoning components.
- Scaling hybrid AI for real-time decision-making applications, such as robotics or fraud detection, requires optimizing efficiency.
C. Interpretability and Trust
- While hybrid AI improves explainability, it still requires better transparency in decision-making.
- Organizations adopting hybrid AI must ensure that their models align with ethical AI principles and regulatory compliance standards.
As research in hybrid AI advances, overcoming these challenges will be key to making AI more versatile, interpretable, and widely adopted.
4. The Future of AI with Hybrid Systems
Hybrid AI represents a major step toward building more human-like intelligence, where machines can learn from data, reason through logic, and explain their decisions. Future developments in hybrid AI may include:
- Advancements in neuro-symbolic AI, leading to AI that combines deep learning with human-like reasoning skills.
- More efficient hybrid AI architectures, reducing the computational cost of integrating symbolic reasoning with machine learning.
- Improved AI interpretability, making AI-driven decisions more transparent and accountable.
- Wider adoption in industries like healthcare, finance, and robotics, where explainability and reasoning are crucial.
By embracing hybrid AI, we are moving closer to creating systems that not only recognize patterns but also understand context, reason through complex problems, and interact with humans in a more natural way.
Final Thoughts: A New Direction in AI Research
Hybrid AI is not just an incremental improvement—it represents a new direction in AI research and development, where deep learning and symbolic reasoning work together to create more adaptable, reliable, and interpretable AI systems.
As industries continue integrating AI into their workflows, the ability to combine learning with logic-based reasoning will be crucial in overcoming AI’s current limitations. The future of AI will not be defined by machine learning alone, but by the seamless fusion of different AI methodologies working in harmony.
The organizations that embrace hybrid AI today will be at the forefront of the next wave of intelligent automation, decision-making, and problem-solving.